Contents
In recent years, Natural Language Processing, or NLP, has evolved the most compared to other Artificial Intelligence (AI) subsets. As the perfect combination of AI and machine learning, NLP applications have greatly transformed how businesses perform fundamental tasks. Particularly, the financial sector is witnessing permanent changes thanks to NLP.
Unsolved challenges for finance service providers
Along with the development of technology, financial organizations have found more methods to increase productivity and create more satisfying customer experiences. More than that, they are getting closer to the end-users: 96% of consumers know at least one fintech service or company worldwide.
The growing market opens more possibilities for businesses, yet it also leads to 3 major problems:
- How to quickly and accurately process a huge database?
- How to ensure data security?
- How to decrease the amount of routine manual work and accelerate the trades at the same time?
Let’s analyze each issue.
64% of consumers worldwide have used one or more financial technology platforms, which means there are more than 4 billion active fintech accounts at the moment. This number is impossible to take care of manually, and financial firms must find alternative solutions to process unstructured data and further assist their performance.
Furthermore, as the nature of fintech, the service providers are holding a huge volume of confidential data from their users, such as the IDs and credit card CVV numbers (Card Verification Value). It’s vital to organize the information and eliminate fraud elements. Each employee needs to have different access permission for better control when unexpected scenarios happen.
Last but not least, another challenge for financial service providers is optimizing their resources for the best efficiency. When the staff is stuck at the desk doing repetitive tasks, it causes a huge loss in revenue, time, and effort since companies cannot maximize the full potentials of their human power.
This is when applying NLP in fintech stands out as the key factor that changes the game entirely.
How is NLP used in finance
Understanding NLP
NLP is a branch of Artificial Intelligence (AI), with the goal of making computers understand and use text and spoken words in the same way as humans do.
For the longest time, NLP development was limited by the limit of datasets available to only a few organizations. However, since Google introduced its Transformer network architecture and the availability of open-source libraries, thousands of companies are leveraging it. NLP has made more progress in the past three years than in any other AI field.
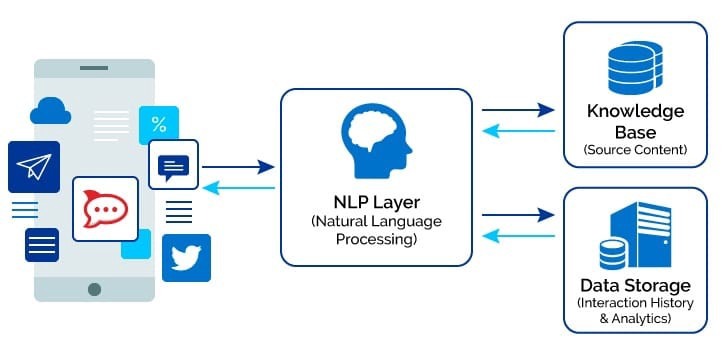
Meanwhile, data is the main driver in the finance industry. And most of the data can be described in the form of text. In the context of finance and banking, users fill in forms to open new bank accounts, and financial experts analyze reports to point out abnormal changes and predict incoming trends, etc.
NLP tools support companies in reading and processing data quicker and smarter. Utilizing the power of machine learning, NLP transforms raw resources into useful insight so companies can save time in processing and analyzing thousands of data gigabytes without breaking a sweat.
As financial institutions and banks work with a massive amount of unstructured data, NLP can be applied in many use cases:
- Sentiment analysis
- Question answering (chatbots)
- Document classification
- Topic clustering
- Text summarization
Therefore, NLP quickly becomes an indispensable ally in the financial sector: From traders, analysts, Fintech CEOs to banks and other organizations are starting to integrate NLP into their system.
Key benefits of NLP as a tool for financial analysis
Efficiency
No one can deny the enormous changes in productivity thanks to optimizing NLP in fintech. With such a small change as integrating a chatbot, you’ve saved hours of work for your support team, which they can focus on more important and complex tasks. “You can apply machine learning pretty much anywhere, whether it’s in low-level data collection or high-level client-facing products,” said Georg Kucsko, an MIT Sloan lecturer in finance.
Speed
NLP is designed as a real-time solution that can provide decisions with supporting data. Rather than taking weeks, now it is only less than a day to finalize a report for a whole region. Moreover, companies can set up the applications to receive results periodically.
Security
As the most important factor to separate outstanding financial providers from other competitors, security is what NLP offers to those who use it. As a spam and fraud detection tool, NLP eliminates the risk that hackers can withdraw information or money from customers’ accounts. It can also encrypt confidential information to ensure that nothing will be leaked to outsiders.
Accuracy and Consistency
It’s merely unreal to avoid human errors when analyzing massive databases manually. A slight miscalculation can cause a huge disaster in the stock market. With NLP, financial firms don’t have to worry about these careless mistakes since it has been programmed to perform the exact formulas every time. More than that, NLP can track a company’s changes over time to predict future scenarios. Therefore, companies can prepare suitable tactics and catch up with the trends.
Rising NLP applications in Finance
NLP applications vary when it comes to customization to fit the needs of each business. Below are the three most prominent use cases of NLP in terms of financial analysis.
Risk assessments
Risk assessments involve determining the likelihood of loss on an asset, loan, or investment. NLP is especially useful when it comes to qualitative analysis, which includes the assessment of a company’s management, vendor relationships, and public perception.
Gathering data and processing unstructured, semi-structured data is the biggest challenge in qualitative analysis. Most analysts must go through news reports, gigabytes of a company’s filings to understand its people, culture, business models, and competitive advantages.
Meanwhile, NLP can be used to parse unstructured documents, extract key information, and summarize text. It can even identity risk with a particular dimensionality reduction algorithm. NLP helps analysts save hours on documents and focus on more strategic tasks.
NLP can also be deployed to help asset managers evaluate investment strategies based on news articles, social media comments, business internal documents, etc. Legal departments can use NLP to analyze financial data, company governance documentation, internal documents, legal text, and contracts. Another use for NLP is monitoring public sentiment about a company.
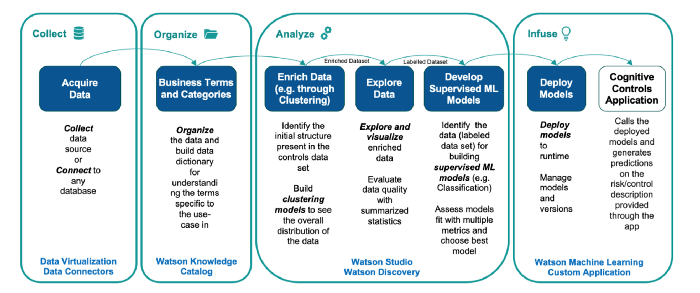
Here’s an example of how the Ernst & Young team supports its bank client in increasing the sales closing rate with NLP. Using semantic pattern matching and sentiment analysis, EY can analyze customer signals and identify cases with a high risk of losing. Thanks to this, the bank achieved a more than 90% capture rate of sales practice issues and a more than 30% decrease in false-positive cases.
Fraud detection
In 2020, there’s a threefold increase in identity fraud attempts in the overall rates year over year in the fintech sector. Businesses are finding more effective ways to tighten their security policies and prevent frauds, violations, and money laundering. NLP can be a big help to make this vision come true.
Based on customers behaviors’ within the fintech platform, developers can label valid actions that identify a legit account. As a filter, NLP can mark out words or phrases that seem unoriginal and fake when one account behaves strangely and alert the business whether this is a fraud or not. These patterns may include an address adjustment, duplicate card requests, password resets, time and place of the payment, and more.
Accounting and auditing
Rather than spending lots of effort to handle endless daily transaction data, financial service providers are turning to NLP to simplify the process. The Big Fours in Finance have already utilized this method.
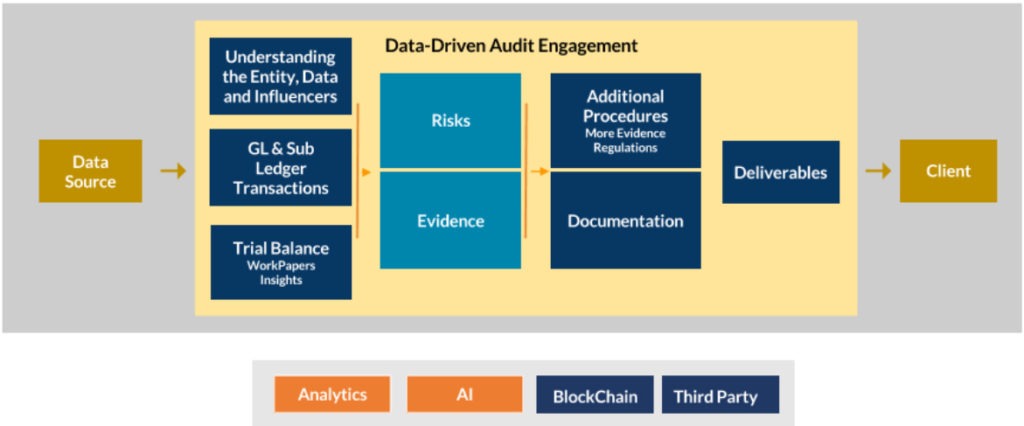
For instance, KPMG in the US analyzes 100% of revenue or purchase transactions through RPA to help auditors make judgments in risk-averse areas, as well as identify potential outliers and exceptions. Then they use NLP technologies to read source documents (e.g. invoices) to compare with transactional records. Thanks to this, auditors can move quickly through transactions and allow more time to focus on critical areas.
Pointing out irregularities also plays a major role in providing thorough audits and long-term strategies. PwC combines NLP and RPA (Robotic Process Automation) to analyze revenue and purchase transactions as well as indicate potential risks and exceptions.
There is also potential for NLP to look and find audit evidence in emails and other documents.
Contract analysis
JP Morgan – the biggest bank in the US, recently deployed a program called COIN – short for Contract Intelligence. This program is a machine learning system that runs on the bank’s new private cloud network. It automates document review for a certain class of contracts.
The algorithm used unsupervised learning by digesting data on the bank’s contracts. It can identify and categorize repeated clauses, classify clauses into different attributes, and note certain patterns based on clause wording or location in the agreement.
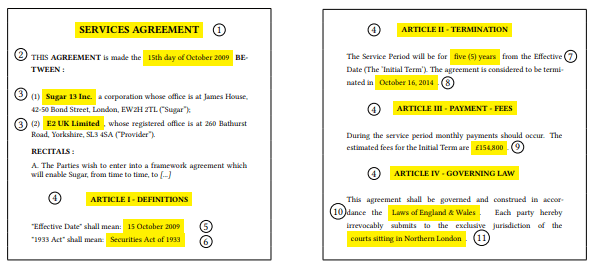
The bank reported that COIN has saved 360,000 man-hours of lawyer and loan officers each year tackling mundane tasks, such as document reviews and interpreting commercial loan agreements.
Financial sentiment analysis
From stock market investment to assets management, NLP-powered sentiment analysis can help process social media streams, financial news, and reports from the financial market to harvest business intelligence. Financial sentiment analysis is an advanced form of sentiment analysis. Not only does it consider the positivity of a piece of information but also how the information will affect the stock market and stock prices.
A notable example is BUZZ Indexes, which is an index fund consisting of 75 large-cap US stocks with the most positive sentiment according to their analytics models. The stocks in this index are selected and scored using machine learning and NLP algorithms, which process millions of data points from social media, news, and blogs. These algorithms bring investors with actionable insights, helping the index to outperform S&P500.
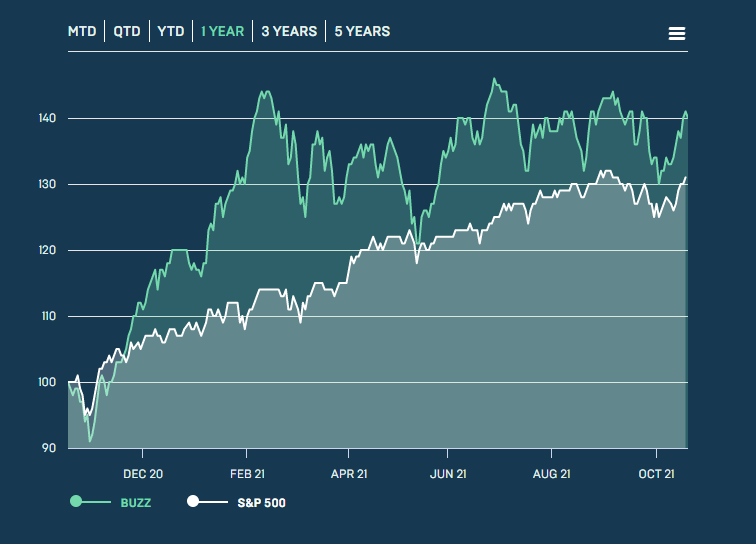
Future outlook of NLP in finance
Many firms have successfully led the revolution for NLP in the financial sector. And this trend shows no sign of stopping in the near future. The technology is now getting closer to more organizations, unlike in the past when it was considered too expensive to implement.
“It’s actually pretty feasible now to do cutting-edge, state-of-the-art NLP in finance, or any domain, without a Ph.D.in machine learning,” said Michael Shulman, a finance lecturer at MIT Sloan.
There are two most common ways for organizations to start applying NLP: First, integrating the technology directly into its system with an in-house team, or second, hiring professional experts to run the whole process.
The finance industry is full of numbers and data; those who understand these goldmines are the ones holding the key to success. That’s why more and more firms are utilizing NLP in fintech to maximize their data processing performance and reduce fraudulent risks. The world is witnessing how AI and machine learning help us stabilize financial status and predict upcoming trends; thereby, we can prepare and adapt to new economic changes better.
Accelerate NLP-driven success in finance with leading IT experts
NLP is actively infiltrating the finance sector and offering unprecedented opportunities for data analysis, customer service, and operational efficiency.
GEM Corporation is one of the best-known IT outsourcing companies among global clients, including Fortune 1000 ones across the EU, the US, and the APAC region. We have received high praises from them thanks to the award-winning IT services, ISO-certified delivery process, and a thriving team of 300+ engineers and experts.
Fill out the form below and let’s explore the possibilities together!
Error: Contact form not found.