Contents
Fraud is getting more sophisticated and costly
Why do we need AI and ML in fraud detection? In a study titled “The True Cost of Fraud” of LexisNexis Risk Solutions, alarming findings surrounding fraud have been pointed out. In 2021, a considerable rise in the costs of fraud was recorded – U.S. retail and e-commerce businesses now lose $3.60 per every $1 of fraud. This figure is 15% higher than the pre-Covid one recorded in 2019 ($3.13). It is also 7.1% higher than that of 2020, as illustrated in the graph below. These losses may come from fines, legal fees, investigation costs, and recovery expenses.
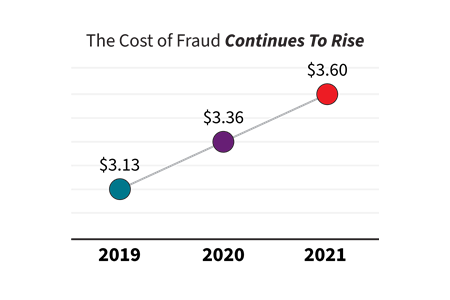
Besides, the study emphasizes several fraud trends before and after COVID-19 in the financial service sector.
- First, the number of successful attacks has surged during the pandemic, most significantly among large institutions. This results in greater financial losses, which stem from the extra work needed for fraud detection and investigation, together with fraud recovery.
- Second, identity-based attacks accounted for most of the fraud-related damage. For mid to large-sized companies, they represent 20% of the losses recorded in 2020. Also, these attacks mostly occurred in the form of account takeovers.
- Third, many companies with online and mobile channel transactions have little or no investment in digital risk mitigation solutions. Lacking such an essential tool to validate activities and transactions may subject them to the risk of detrimental breachers.
With such detailed insights into how frauds continue to trouble businesses, the study has indicated the urgent need to effectively detect and prevent fraudulent activities. One of the ways to tackle this is leveraging AI and ML in fraud detection.
AI and ML in fraud detection and prevention
AI refers to the more general application of analytics to create simulations of human intelligence and accomplish tasks. ML is its subset which focuses on enabling machines to learn from datasets and make intelligent decisions automatically.
The most important benefit of using AI and ML in fraud detection to be highlighted is efficiency. ML determines fraudulent activities by analyzing current patterns and spotting abnormalities from a large number of streaming transactions. This process can be completed more quickly and with greater precision than human analysts, allowing real-time approval of transactions and timely detection of any potential problem. Besides, the technology’s highly accurate predictions can eliminate false positives and human errors.
Another advantage of using AI and ML in fraud detection is scalability. ML-based methods become more refined over time as they come in contact with more data. This ability to self-evolve is key to help businesses achieve long-term growth.
Fraud detection models and their use cases
Leveraging AI and ML in fraud detection creates multiple models that can be divided into two categories: binary classifiers and anomaly detectors, with different applications for varied aspects of fraudulent activities. Each category will be explored through its most prominent example – respectively Logistic Regression and Isolation Forest.
Logistic Regression
Logistic Regression predicts the probability of a class or event with a score from 0 to 1. When it comes to AI and ML in fraud detection, it has proven to be reliable for spotting email phishing and filtering unwanted spam from large bulks of emails, which is an important aspect of fraud prevention. Take Phishing emails, spam letters with fraudulent purposes, for example. Usually, phishers will create fake websites and URLs similar to those of banking firms, convincing users to follow their instructions. Users may be asked to provide sensitive information or make payments.
Generally, the models will be trained by being exposed to a number of similar malicious and legit emails. Afterward, they will apply that knowledge to classify abnormal inputs. Variables possibly associated with spam emails that are used for the training phase are listed in the table below:

In a research paper, an email classifier built on this basis demonstrated an impressive accuracy rate of more than 98%.
Isolation Forest
Another example of using AI and ML in fraud detection is Isolation Forest models. They facilitate the process of anomaly detection. In simple terms, the goal of an Isolation Forest is to separate the “outliers.” The premise of this algorithm is that through random data partitioning, anomalous points are easier to be isolated compared to regular ones.
Meanwhile, spotting abnormal activities and patterns is critical to prevent identity theft, which, as mentioned above, is the most prominent type of fraudulent activity. For this task, the models in use will focus on creating a database of one user’s behavioral patterns and constantly comparing them to the current activities. If there is a significant difference, the system will suspect the account has been stolen and alert all parties involved. Therefore, it further proves the great potential of leveraging AI and ML in fraud detection.
Isolation Forest has been proven by many research papers to be a highly intelligent and effective solution to detecting identity fraud. For instance, an experiment was carried out to demonstrate the use of Isolation Forest to identify credit card fraud. Compared to the Local Outlier Factor algorithm, the Isolation Forest one has a higher accuracy rate of 99.5%.
How can businesses successfully implement AI and ML in fraud prevention?
If applied properly, AI and ML in fraud detection will offer substantial assistance in recognizing fraudulent behaviors while also continuously adapting to new fraud tactics. Otherwise, without sufficient understanding and preparation, businesses can suffer from poorly architected systems that make costly mistakes and hinder decision-making.
According to Forbes, firms should keep in mind the following matters to design fraud detection strategies powered by AI and ML:
- Data accuracy: Testing and validating the models on a regular basis will guarantee that the datasets are free from noise, missing values, and outliers. This practice is, therefore, crucial for accurate outputs.
- Data bias: When a data bias is present in an ML model, certain elements are more heavily represented than others. A biased dataset will lead to skewed outcomes with lower accuracy levels and little analytic value.
- Defined goals and objectives: This might seem obvious, but businesses need to have a detailed, fraud-specific plan when applying AI and ML in fraud detection. They need to ask questions such as what problems they want to solve, how they should allocate their resources, and what specific use cases will maximize ML’s values for their organization.
Closing thoughts
In a fast-moving world, companies in the finance industry are constantly challenged by fraudsters and their many tactics to infiltrate systems. As a result, they are strongly advised to leverage tools powered by AI and ML in fraud detection to unlock a tech-driven approach.
Harness the power of AI and ML in fraud detection and more!
As one of the pioneers in ITO with award-winning services and CMMI-appraised and ISO-certified processes, we have successfully implemented over 100 projects, delivering robust solutions across diverse industries, including BFSI.
With an extensive portfolio containing key BFSI-related services like building payment portals and integrating banking services, GEM is here to help you make a difference.
Fill in the form below and we’ll get back to you as soon as we can!
Error: Contact form not found.